Anomaly Detection on Steel Surfaces
.png?width=1000&height=750&name=Rail%20(Custom).png)
.png)
Machine learning helps steel surface inspection
In the past, it has been difficult to inspect vibrant or vivid materials like steel, wood, and fabric for anomalies.
It is extremely difficult to design a traditional, feature-based algorithm to detect anomalies in such materials.
Often a traditional algorithm would have flaws, like too high a false reject rate or a low detection rate on certain types of defects.
With machine learning, this can be trained to a network, based on a large annotated sample set. A network is trained with both anomalies and acceptable sample images.
The optimum state of the machine learning network is when the network is capable of generalizing and thereby detecting anomalies in unprecedented images.
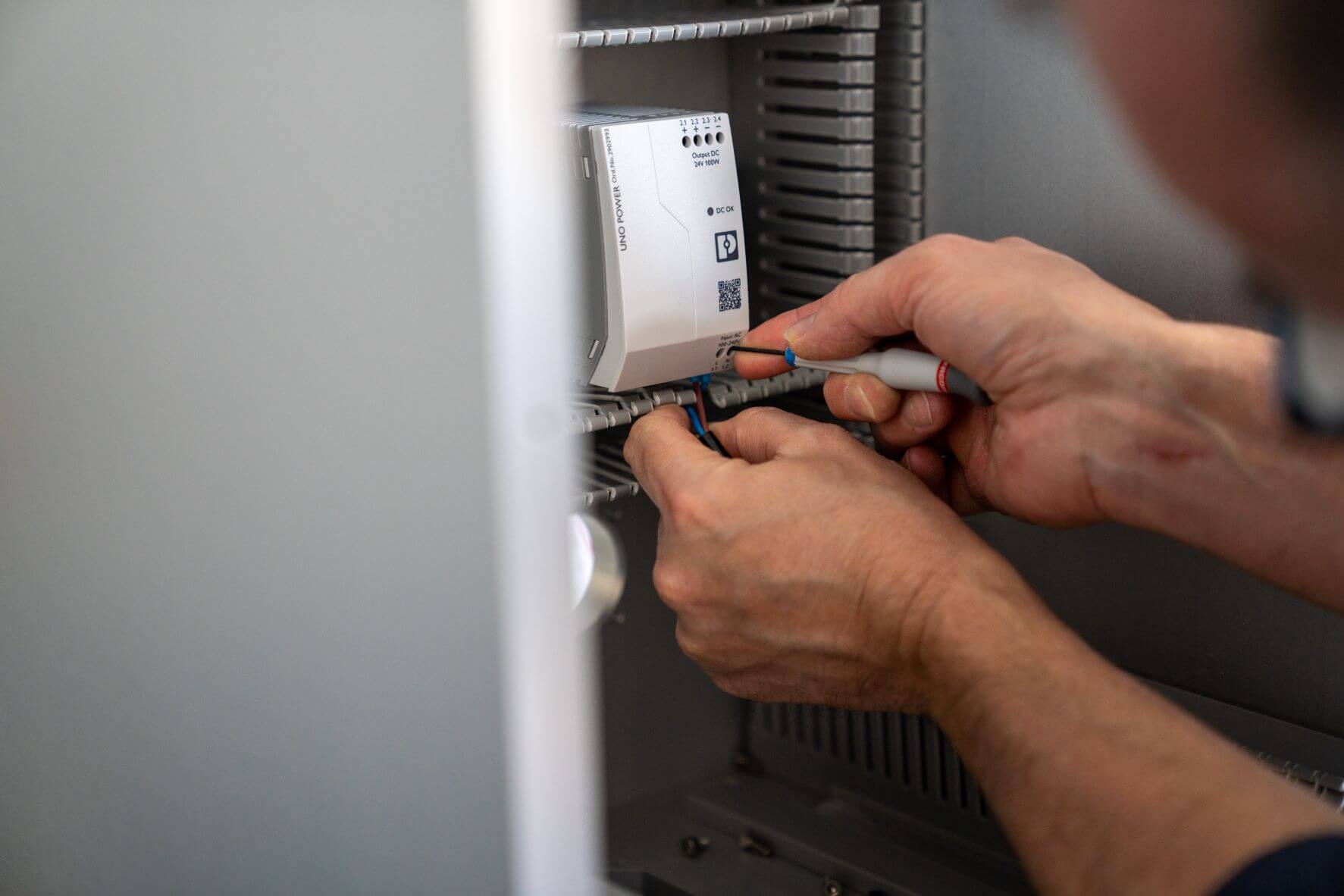
Replacing a labor-intensive manual inspection process
The JLI Rail Surface Inspection System is an example of a system that replaces a process that previously required a number of people to visually evaluate each image for anomalies.
This job requires a high level of focus and can be very cumbersome.
A machine learning solution on top of the Rail Surface Inspection makes a lot of sense when it comes to robust, repetitive inspections on the fly.
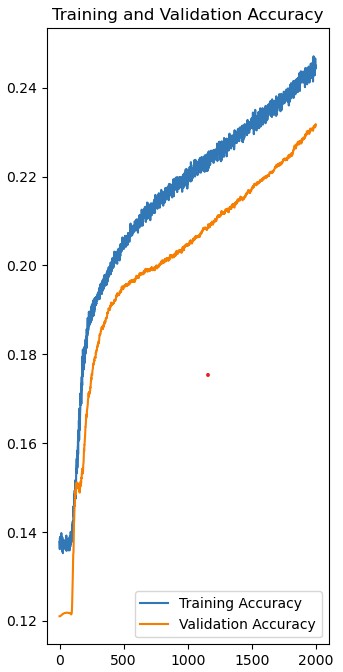
A network capable of handling a large dataflow
JLI designed a network that could work on the output from the Rail Surface Inspection System.
The network was trained on a large number of annotated anomalies and tested on +10.000 images to ensure a low false reject rate and a high true detection rate.
It is of great importance that a machine learning network is able to handle the large data flow – in this case, live stream pictures from 6 megapixels cameras.
The hardware design utilizes a GPU to accelerate the algorithm locally. It can be scaled with more GPUs if required.
JLI has chosen to execute the machine learning network locally to avoid any latency in execution.
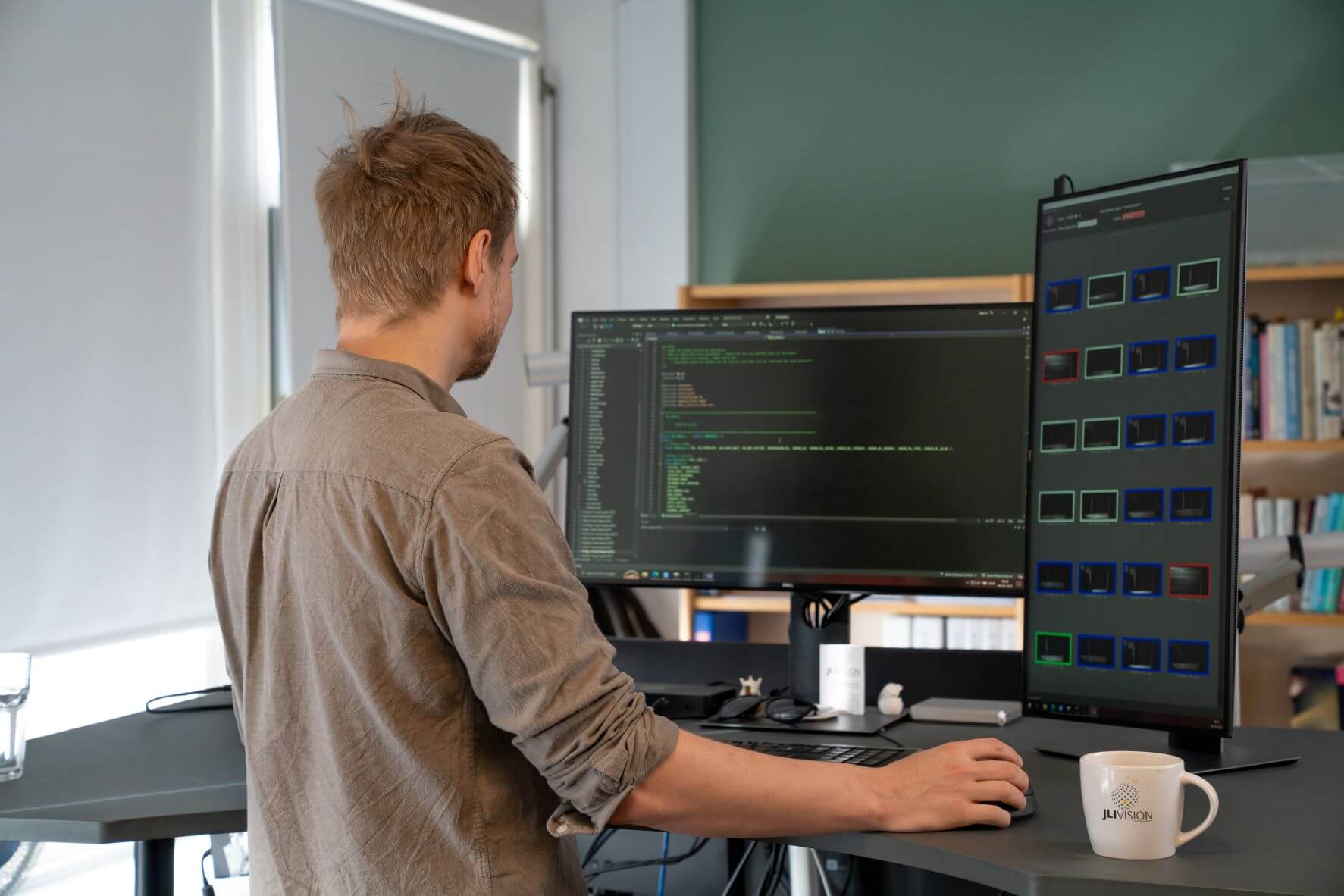
A detection rate above 95%
The detection rate of the system is >95% which is better and more consistent than the previous manual inspection.
Defective images are stored for review by an operator, who can decide how to handle defects.
An early warning signal can be raised, when recurring process-related defects are detected.
This feature enables the operator to use the Anomaly Network as a tool to improve production yield and gain a higher throughput of good objects.
Detection accuracy is >95% which is better and more consistent than the previous manual inspection.
.jpg?width=1440&height=696&name=Group%2050%20(1).jpg)
Download the case study
Can we help you, too?
.png)
Send me an email at hb@jlivision.com
or book a meeting, and let's find out how we can help you.
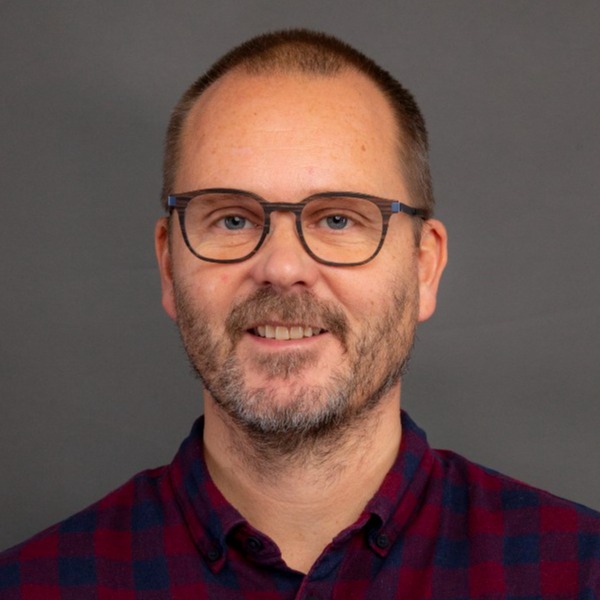